Consumer vs Industrial
Joshua Bloom reminds us how the impact of false positives in machine learning in the consumer vs industrial world are different.
In the consumer space, a false positive may accidentally send you a shirt you don't like, or a false negative may fail to send an advertisement. In healthcare, a false positive may accidentally suggest a patient requires urgent surgery, and a false negative may be fatal.
Four Quadrant Exercise
This four quadrant exercise outlines the impact of true positives & negatives, and false positives and negatives. It can help articulate in a more explicit manner the potential impact of unintended consequences.
The exercise could be run multiple times, each time imaging a different potential 'scope' of impact for a machine learning project. This can help identify which four quadrant iteration has the most suitably balanced risk profile. In turn, that can be used to set the product's requirements & specifications.
The exercise can also be done prior to implementation of a new product into a workflow to determine potential unintended consequences of false results.
There certainly will be situations where a product may have the potential for incredible true positive and true negative results; however, the false positive and false negatives that result from it are unable to be satisfactory mitigated and lead to favouring a more 'restrained' implementation.
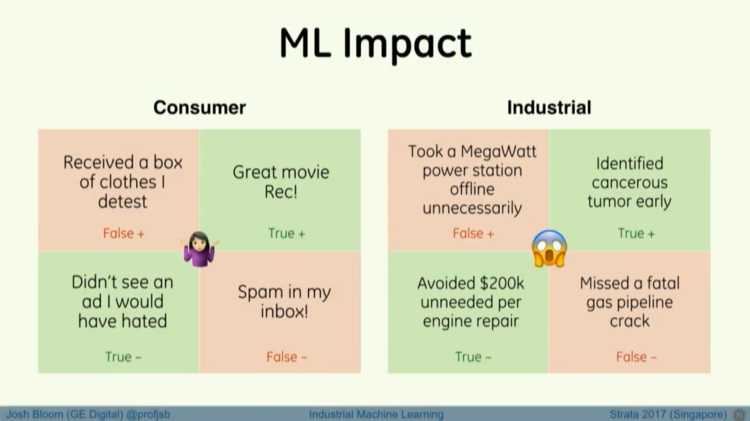
As always, the best book on the impact of technology on life, and the importance of unintended consequences is Ursula Franklin's The real world of technology.